A recent retrospective study led by Dr. Marilyn J. Siegel and her team at the Washington University School of Medicine in St. Louis has shed light on a critical issue in cancer care: routine clinical reads are more prone to overdiagnosing progressive disease when compared to RECIST 1.1 interpretations. This discrepancy holds significant implications, potentially leading to the premature discontinuation of effective treatments for cancer clinical trial participants and patients under standard care.
In this study, mint Lesion software was utilized for the criteria-based reads, determining overall response assessments according to RECIST 1.1 criteria, and generating structured reports for the clinical trial's principal investigator.
To learn more about the study's insights into the discrepant assessments and the suggested steps for mitigating this issue, click here.
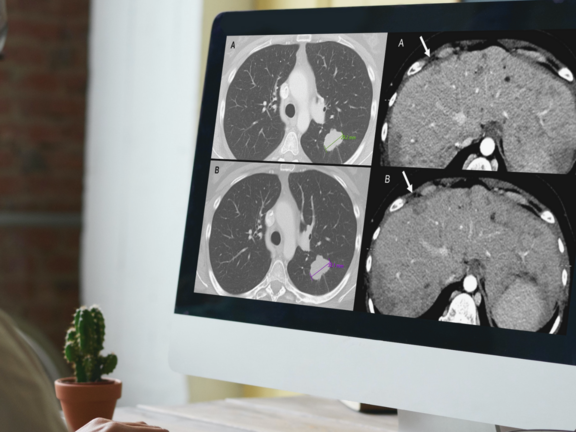
Study Discovers Overdiagnosis of Progressive Cancer in Routine Clinical Evaluations
Related Resources
Related Resources
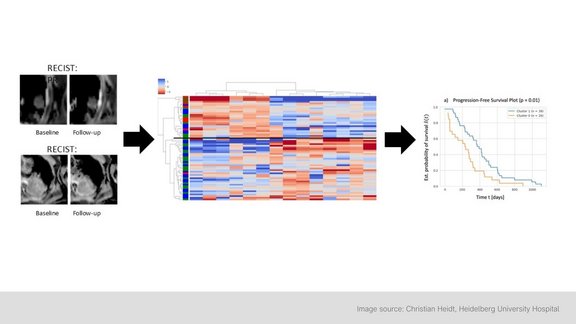
Heidelberg University Hospital: Delta-Radiomics Features from ADC Maps as Early Predictors of Treatment Success in Lung Cancer Therapy
In this prospective study conducted by Heidelberg University Hospital, researchers investigated whether changes in radiomic features from…
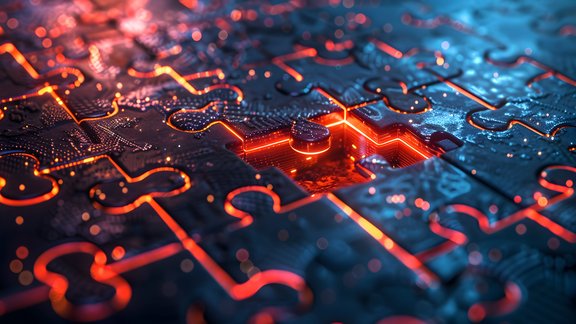
Healthcare on FHIR: Igniting the Potential of Interoperability
Interoperability plays a crucial role in healthcare: it enables seamless communication of patient information across different systems, leads to…
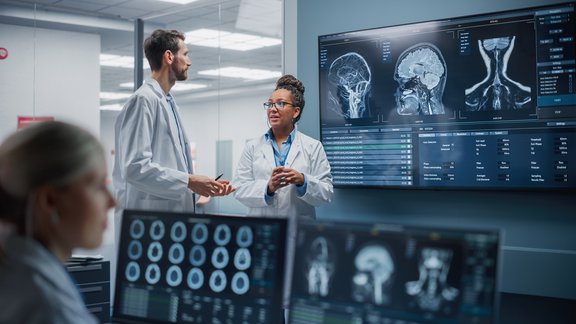
Optimizing Glioblastoma Imaging: Enhancing MRI Efficiency and Quality with Deep Learning
This study investigates the use of deep learning (DL) to optimize MRI protocols for glioblastoma patients, aiming to reduce scan time and improve…