Advances in technology, especially in the field of AI, can significantly relieve the ever-increasing workload of radiologists today. We are continuously striving to make the most out of the countless possibilities AI offers. Therefore, we are about to incorporate multiple AI elements into mint Lesion™ with various partners and an example of this is an upcoming plug-in, with which it will be possible to automatically detect and 3D-segment the prostate in MR T2-weighted images.
The new plug-in, Prostate.Carcinoma.ai from our partner FUSE-AI, will simplify and speed up prostate screenings according to PI-RADS 2.1. At the touch of a button, a full segmentation of the prostate will be calculated within mint Lesion™, including findings of benign or malignant lesions. By using the automatically calculated volume segmentation of the prostate, mint Lesion™ automatically maps the lesions to the prostate lesion scheme, making manual drawings unnecessary.
In the early development phase, the plug-in was made available to Dr. Alexander Cornelius and his team at the Cantonal Hospital Aarau to test and provide user feedback. Dr. Cornelius states: “AI in radiology is the logical consequence of the ongoing developments in recent years to reach a new level of medical and clinical care. For me, even the first results from the Prostate.Carcinoma.ai plug-in in mint Lesion™ were a substantial improvement!“
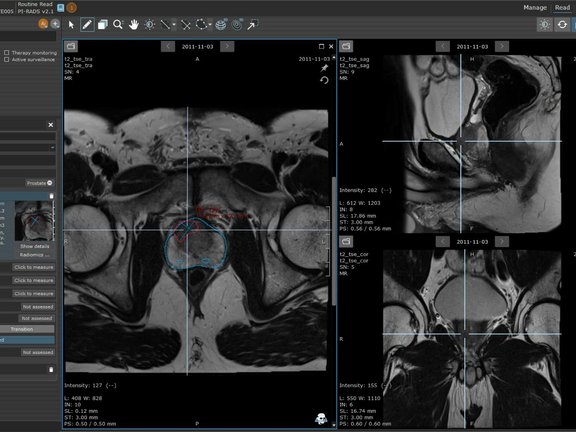
Fighting prostate cancer with AI and automatic segmentation
Related Resources
Related Resources
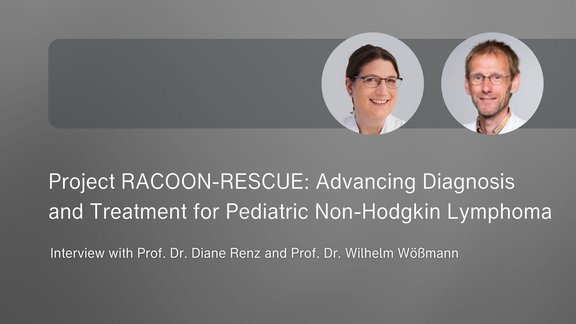
Project RACOON-RESCUE: Advancing Diagnosis and Treatment for Pediatric Non-Hodgkin Lymphoma
Pediatric Non-Hodgkin Lymphoma (NHL), a type of lymph node cancer, is the fourth most common tumor in children and adolescents. Radiological…
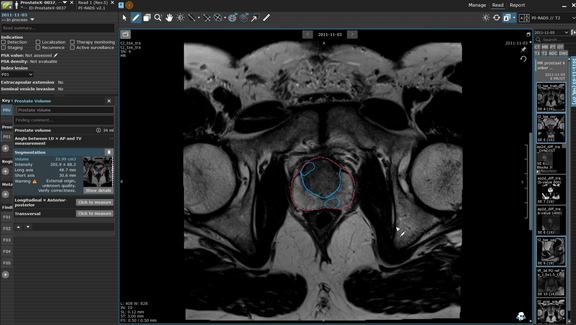
Streamline Prostate Cancer Screenings with Prostate.Carcinoma.ai in mint Lesion
The Prostate.Carcinoma.ai plug-in, developed by our partner FUSE-AI, is a powerful addition to mint Lesion, designed specifically to enhance prostate…
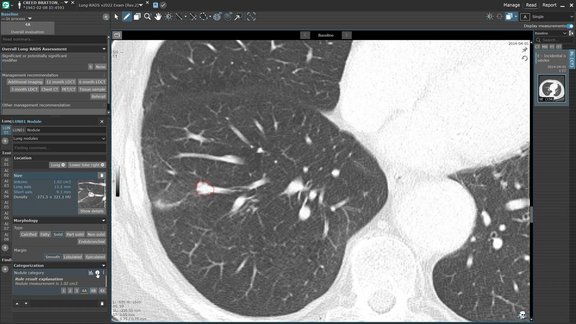
Leverage Advanced AI-Driven Nodule Detection and Analysis for Comprehensive Patient Care
Discover the power of streamlined AI-driven lung screening with contextflow ADVANCE Chest CT integrated into mint Lesion. With automated lung nodule…