Funded by the Ministry of Science, Research and the Arts of Baden-Wuerttemberg, Mint Medical has been supporting the Heidelberg University Hospital as a cooperation partner in an innovative project since March 2020: the development of a "cognitive medical assistant (KoMed)" that can identify a patient's individual surgical risk in advance, facilitate therapy decisions, and prevent complications.
According to the German Federal Statistical Office, approximately 17 million surgeries [1] are performed in Germany every year. While the majority of patients benefit from surgery, there are also those who suffer complications during or after the procedure. To date, risk scores have been determined according to criteria such as age, gender and previous illnesses, but these do not adequately reflect the actual risk of complications for the individual patient. In order to minimize complications and take potential risks into account before surgery, an interdisciplinary team from the Department of Anesthesiology and the Department of General, Visceral and Transplant Surgery, the Institute of Medical Biometry and Informatics, the Department of Medical Information Systems and the Center for Information and Medical Technology will train an algorithm over the next two years to evaluate a large amount of clinical data from patients using Big Data analyses. This will allow KoMed to provide informed decision support for adapted treatments by machine-learning which features are more or less likely to lead to complications such as wound infections or heart attacks. "This not only gives patients and treatment teams more certainty when making treatment decisions," explains project leader Dr. Jan Larmann, senior physician of the Department of Anesthesiology at the University Hospital, "assessing risks as accurately as possible also allows resources to be used in a targeted manner and thus also brings economic benefits."
As part of a clinical trial starting off with around 600 patients, routine data and treatment histories are initially processed in a structured and analyzable manner so that KoMed can learn to identify risks as accurately as possible. Mint Medical supports this data collection with mint Lesion™ and eCRFs (electronic case report forms) developed specifically for the project, which make the data available in a standardized and traceable form. In addition, dashboards optimized specifically for the project are set up in mint Analytics, an add-on of mint Lesion™, which can be used to monitor the status of the clinical trial in real time. With the data systematically collected through clinical routine and additional proteome analyses, the project team expects to gain a better understanding of the circumstances under which complications occur: "This will make it possible to implement targeted countermeasures in the future," asserts Dr. Larmann confidently.
Watch the video here. [Video in German]
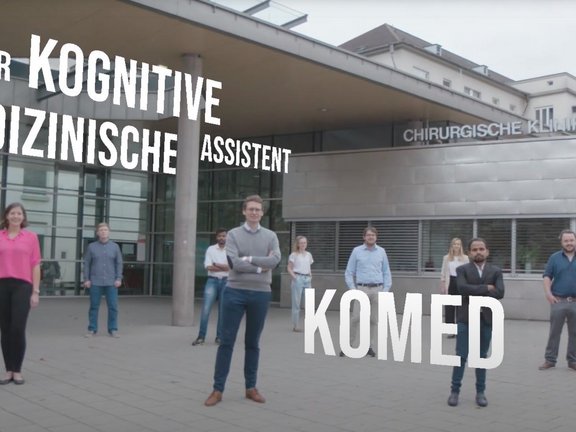
Project „KoMed“: The cognitive medical assistant for risk and complication prevention
Related Resources
Related Resources
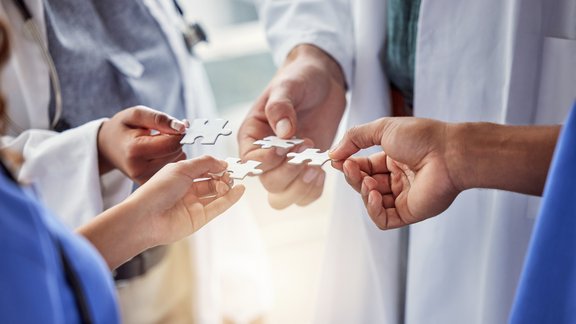
Developing Standardized Reporting Templates for Sarcomas: Insights from Prof. Dr. Wolfgang Kunz
Sarcomas are a rare and heterogeneous group of malignant tumors that pose significant challenges in both diagnosis and treatment. Within the framework…
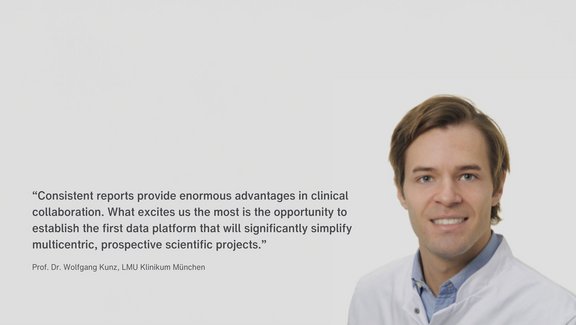
Standardized Reporting Template for Sarcomas in the BZKF BORN-Project: Interview with Prof. Dr. Wolfgang Kunz of LMU Munich
Sarcomas are a rare and highly diverse group of malignant tumors that affect both soft tissue and bones, posing significant challenges due to their…
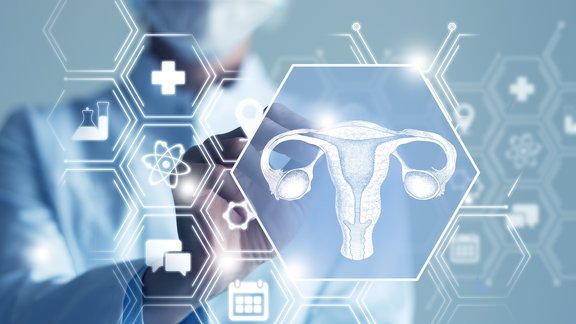
RACOON FADEN Project Tackles Early Detection of Adenomyosis
Endometriosis is a vastly under-researched condition affecting women, but it is finally receiving the attention it deserves through the RACOON FADEN…